Big Data in Modern Computational Toxicology
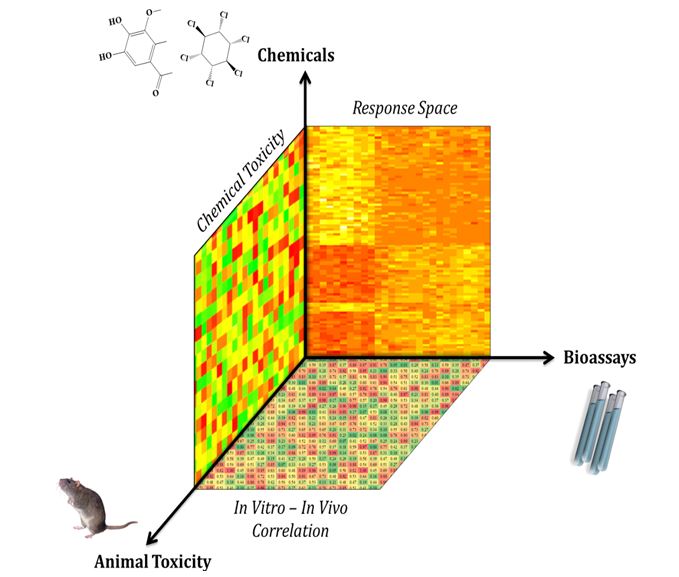
Leader: The big data approaches developed in this project are expected to directly evaluate the chemical animal toxicity for new compounds from existing public toxicity data. Additionally, the chemical-in vitro-in vivo relationships developed in the modeling process can be used to explain and predict potential toxicity mechanisms.
Figure 1. The chemical in vitro-in vivo relationships in the toxicity big data landscape.
Summary: High Throughput Screening (HTS) assays that measure the in vitro (in the test tube) toxicity of environmental compounds have been widely applied as an alternative to in vivo (in living things) animal tests of chemical toxicity. Current HTS studies provide the environmental and toxicological scientific community with rich toxicology data that has the potential to be integrated into toxicity research. Furthermore, although the research of toxicity pathways has revealed some promising receptors as potential targets of chemical toxicants, most of the mechanisms of animal toxicity are still obscure. A general question raised from the current big data era is whether and to what extent experimental data obtained from in vitro assays enrich our ability to predict and explain f more complicated toxicity phenomena (e.g. animal toxicity). To address this question, the goal of this project is to use a single assay data as the probe to automatically search and reveal all the relevant information in the public big data pool. All the relevant toxicity data, including the original assay data, will be integrated differently based on the chemical features relevant to the target animal toxicity using advanced data-driven computational approaches. The resulting computational models based on chemical-in vitro-in vivo profiles (CIIPro) will not only be used to predict the animal toxicity of environmental compounds, but will also illustrate the value of developing single or multiple toxicity assays as alternatives to the current use of animal models in the current big data era.
Figure 2. CIIPro website http://ciipro.rutgers.edu/
Next Steps: Currently the CIIPro web portal (ciipro.rutgers.edu) is being developed in Dr. Zhu’s lab with help from Dr. Welsh. The predictions from hybrid models are being experimentally validated in Dr. Aleksunes’ lab. The goal of these efforts is to share the experimentally validated computational toxicity predictors with the environmental and toxicology community.
Investigators:
Hao Zhu, Ph.D., Principal Investigator
William J. Welsh, Ph.D., Co-Investigator
Lauren Aleksunes, Pharm.D., Ph.D., Co-Investigator
Read the published research:
Zhu H, Bouhifd M, Kleinstreuer N, Kroese ED, Liu Z, Luechtefeld T, Pamies D, Shen J, Strauss V, Wu S, Hartung T Supporting read-across using biological data. ALTEX. In press.
http://www.ncbi.nlm.nih.gov/pubmed/26863516
Kim M, Huang R, Sedykh A, Zhang J, Xia M, Zhu H* Mechanism profiling liver toxicants by using antioxidant response element assay data model and public big data. Environ. Health Perspect. In press.
http://www.ncbi.nlm.nih.gov/pubmed/26383846
Zhu H*, Zhang J, Kim M, Boison A, Sedykh A, Moran K Big data in chemical toxicity research: The use of high-throughput screening assays to identify potential toxicants. Chem. Res. Tox. 2014; (27) 1643-1651.
http://www.ncbi.nlm.nih.gov/pubmed/25195622
Zhang J, Hsieh J-H, Zhu H* Profiling animal toxicants by automatically mining public bioassay data: A big data approach for computational toxicology. PLoS One 2014; (9) e99863.
http://www.ncbi.nlm.nih.gov/pubmed/24950175
Funding:
This research was funded in part by the National Institute of Environmental Health Sciences (NIEHS) through the Center for Environmental Exposures and Disease at EOHSI (NIH-NIEHS P30 ES005022) and NIH-NIEHS R15ES023148.